Application of artificial intelligence in manufacturing sector in India is presently growing at a compound annual growth rate of 49.5%. The market of the manufacturing industry is expected to be evaluated at $1 billion in 2019. It is forecasted to grow up to $17 billion by 2025. These figures paint a vivid landscape of definite need and an ultimate scope for any manufacturing business with AI.
Infrastructural Needs of Artificial Intelligence In Manufacturing
Hardware
Computational ability is a massive requirement of increasing applications of artificial intelligence in the core manufacturing sector. Infrastructure finds high computational load due to artificial neural networks, machine learning algorithms, and big data analytics tools. It is generating a genuine need for AI for powerfully impacting the manufacturing sector.
High-end computational units in global scale manufacturing industries are also looking for IOT-based cohesive networks. They help them generate continuous streams of data points, significant data routines, and analytics regime. There are critical requirements of AI integrated bots in production lines and holistic support around operations at all times.
Top example: Shell global solution powered by Microsoft for IoT
Software
The first and foremost requirement is to build optimization machine learning models that increase efficiency and overall profitability of a manufacturing business. Machine learning models can excellent-tune target variables to reduce time material cost. It also increases power usage effectiveness (PUE), and critically shoot in-house resource utilization for maximized output.
The successful intrusion of AI in the manufacturing sector also underlines the need of analytics software and database integrated dynamic supply chain management (SCM) solutions, integrated enterprise resource planning (ERP) management and well-built CRM solutions. The trio of supply chain management solutions, CRM, and ERP are essential for capitalizing on a broad scope for AI. Successful inclusion of artificially intelligent modules enables you with:
- Interactive UX in complex ecosystems
- Descriptive, predictive and prescriptive analytics
- Advanced BI
- Automated monitoring
- Controlled operations
Top example: SAS for manufacturing
AI-based SaaS Products For The Manufacturing Sector
There lies a vast business opportunity set up within the product manufacturing sector. SaaS products are currently on an upstream of market capturing, and there are definite trends of businesses acknowledging their presence. Dedicated, niche SaaS products for the incorporation of AI in manufacturing-related digital systems allow:
- Micro retail-based products
- No in-house complications
- Reduced development and maintenance cost
Technological Needs Of Artificial Intelligence In Manufacturing
Industrial Robots
Industries like automobile, electronic, and hard welding have always faced a shortage of labor and industrial robots have powerfully countered the considerable problem. Robots have a direct effect on average ROI and optimal resource utilization of finance and investments. Artificial intelligence help playing a massive role in making companies magnify the internal efficiency for reducing the external dependencies on business entities.
Top example: Siemens has developed a path-breaking unmanned aerial vehicle (UAV) that is powered by IOT and AI for fault detection in long transmission lines.
Quality control
Quality assurance in manufacturing lines of large-scale production industries should be visualized as a data-driven process on the work terminals. These automated processes are governed by various quality checking algorithms, set patterns, and pre-set guidelines/parameters, well monitored by policing and inspecting sensors. All the data collected by sensors and predefined programming of AI algorithms govern top-notch quality assurance activities in manufacturing businesses. It is a massive opportunity for companies to reduce their loss due to wastage and increase the level of precision in bulk manufacturing tasks.
Enjoying this article so far?
You’d also enjoy reading: Investor Confidence In India: Trends Over The Years And Future Projections
Top example: Domino’s Pizza checker is an artificial intelligence tool that inspects photographs of every pizza delivered. It checks if it is having all the ingredients and is presented the way it should be.
Cybersecurity
Cybersecurity is of utmost importance for all companies operating in the manufacturing space. These companies have general business processes well embedded in the data-driven tasks. Major cloud compliances and data protection guidelines like GDPR are extremely important. AI plays its part in the manufacturing sector by helping them streamline the hybrid cloud strategy for storing data, and identifying tasks which belong to a day-to-day category and the ones that are unusual and require inspection.
There is a single channel of data flow that requires ultimate interoperability for various data views and integrated analytics for all departments of a manufacturing company. Cybersecurity is extremely important for isolating data related to different departments and their complete security from external threats and malicious attacks.
Top example: Logrhythm, a Colorado-based company that is using machine learning for finally handpicking situations of compromised data, and protected account sand profiles that cause undetected threats.
Maintenance & Inspection
Machine learning is capable of hosting predictive and forecasting solutions based on the present performance data and fine-tuning the target variables for futuristic equations. These equations include factors like external support, load, and a general slowdown in peak performance.
Manufacturing companies can use AI to reduce downtime because of malfunctioning manufacturing machines and secondary resources. Machine learning is capable of predicting instances of failure and complete breakdown by estimating the total life and of any device. Companies can prepare beforehand for such unforeseen situations and avoid a pitfall on the road of maximized resource utilization.
Inspection is best empowered by computer vision that is capable of inspecting the ready products. It also uses artificial intelligence for tracking down the images of products below compliance and manufacturing standards.
Top example: NanoNets is an excellent example of machine vision-based products and ready to use APIs with your integrated manufacturing systems for maintenance and inspection.
Procurement & Planning
Use of AI in procurement and planning leads to synchronized plan with the help of digital supply network (DSNs) that are powered by advanced technologies and interconnected systems. Companies are looking to analyze sales patterns around a year using time series analysis and sentiment analysis. It is done through various data points related to their marketing and client-facing areas.
Using artificial intelligence for such objectives requires critical analysis of point-of-sale data that helps the company design, plan, and source external suppliers. It is supported in making and delivering high-quality products with ultimate profitability and reduced effort.
Top example: There are AI-powered niche platforms that are automating the procurement process. It also comes with an acute forecasting system based on your seasonal sales patterns.
Scope Of Applications Of Artificial Intelligence In Manufacturing Across Various Industries
Automobile
The automobile sector has a genuine need for automation at ground level. Indian automation sector is presently undergoing a critical phase of recession where products worth INR 35,000 crore are currently being shelved looking for buyers. Despite this, there are genuine use cases of AI in automotive.
Machine learning, leading to artificial intelligence is the torchbearer of all autonomous driving projects at the industry level. All major automobile manufacturers have pledged to eradicate fossil fuel-powered vehicles latest by 2030.
There is also a trend of Internet-connected vehicles and internal communication vehicles. The automobile industry is also looking to come up with a mobility-as-a-service concept that requires AI for location-based data analytics. It is a solution to carpooling, vehicle sharing, and other greener practices as are necessary for the eco-friendly automobile industry.
Major Requirements:
- AI for autonomous driving
- IoT-powered AI module for inter-vehicle communication
- A comprehensive solution for mobility-as-a-service
- Smart manufacturing digital life processes
- Inspection of produced vehicles through computer vision
Energy And Power
The power & energy sector can undergo a massive transformation with AI-powered software that helps in intelligent energy management and storage. In-depth analysis of the production-consumption pattern is essential to build sophisticated software that bears renewable energy with AI-driven storage. The next level application of AI enables building interim storage systems, and there is a scope of further optimization of these standalone systems depending upon the commercial or domestic use narrative.
AI can help cultivate conscious use of electricity. Companies can build customer profiles of power consumption and help them analyze the scope of energy consumption reduction. It will help in reducing the overall energy requirement and also make energy-saving decisions based on the production forecast from ML models and production-consumption data.
Major Requirements:
- Energy profiling of customers
- Optimization standalone systems
- Intelligent energy storage systems
- Energy production forecasting and data-driven decision making
Pharmaceutical
Presently, the pharmaceutical industry is looking to combine the power of blockchain-based comprehensive solutions with end-to-end AI capabilities. The objective is to eliminate all possibilities of drug counterfeiting. Manufacturing industries also reportedly use artificial intelligence for BI purposes and streamline their efforts for market acquisition. Visual & design intelligence is also helping in drug design and development based on the 3D arrangement of atoms and molecules through material science.
More on The Brief: Sustainable Manufacturing And Remanufacturing In India: Are We Ready For The Change?
The pharmaceutical industry is marching past 2020 with gradually maturing solutions that are capable of predicting medicinal capabilities impacting the high-level, commercial clinical trials. Moreover, people are looking to use artificial intelligent anesthesia systems for enhanced surgical robotics in close relation with the pharmaceutical industry. Interestingly, USFDA can be taken as an inspiration. It had granted special approval for commercial testing such systems.
Major Requirements:
- Automated anesthesia systems
- Clinical trial outcome prediction
- Medicine capability production
- Suggestive mobile platforms for medicine
Heavy Metals & Machinery Manufacturing
Heavy metal and machinery manufacturing industry is looking to incorporate artificial intelligence for procurement planning, efficient CNC operating modules in utilizable machinery designs based on specific requirements and constraints. Automation of the designing and manufacturing process with complete compliance with 100% scalability is essential for higher profitability.
Major requirements:
- Precise automation of CNC infrastructure
- Suggestive design models
- AI-driven engine for 3D printing and modeling
Semiconductors & Electronics
Semiconductor and electronics industry is facing an acute shortage of skilled laborers, and artificial intelligence is the technology coming to the rescue. The requirement of skilled labor in the Chinese semiconductor electronics manufacturing industry is expected to double from 2017 to 2025. The large-scale condition of highly precise electronic manufacturing lines is being created by artificially intelligent, utterly automated action lines.
The overall chip size of electronic components drastically reduces with time. Precise soldering and laser printing of microchips will require artificial intelligence capabilities and optimization modules. This industry also needs computer vision very badly for improving the overall skills in quality control side.
Major Requirements:
- Automation to fight labor shortage
- Micro, nano-level chip printing
- Suggestive engines for electronic designs
- Inspection and QA with computer vision
Food & Beverages
Continuously growing food tech start-ups are underlining the scope of AI-attention in food and average industry. The food and beverage industry is undoubtedly looking to eliminate the requirement of human labor and decrease the overall human intervention in models.
Foodtech is also brewing AI-enabled suggestive platforms that perform reverse engineering through computer vision on images of food items and tell the requirement of ingredients and raw material. It also suggests a recipe based on the availability of limited parts and raw material.
Major Requirements:
- Automation work for industry
- Recipe suggestion introduction
- Innovation in food items
- Automation and predictive capabilities of supply chain
- AI-enabled check for large-scale food and beverage industry compliance
Significant Challenges For Artificial Intelligence In Manufacturing
Inaccuracy, Maintenance And Cleaning Of Data
Raw data lies in the heart of artificial intelligence and AI-powered solutions. Irrespective of the industry in which artificial intelligence is used, inaccuracy of data and wrongly collected data points are a huge challenge. It directly leads to wastage of time, material cost and inefficiency of AI models targeted at an industry.
Another issue is the maintenance and cleaning of raw data. Data science is an in-demand skill and data scientists who can help you manage truckloads of data increasing at an exponential rate generally require a fortune as a payout. Another issue is that data-driven practices are costly, and they need consistent long-term efforts for substantial benefits.
Deployment Constraints
The core problem of artificially intelligent models is that it runs on the edge while everything else rests on the cloud. There is a massive problem in the deployment of AI-powered models across all manufacturing industries. Top-notch computational ability is required on-premise, which is only possible on the cloud side. Further, all the data and other resources are also resting at the cloud, and while AI requires on-site computational capabilities.
Efficient SaaS products dedicatedly built for particular manufacturing industry can help resolve this problem.
Shortage Of Technically Skilled Personnel
According to monster.com, the most in-demand skills in the present day are machine learning, artificial intelligence, and natural language processing. It is often seen that a plastic intelligence model requires machine learning artificial neural networks and high-end analytics. The skills are facing a scarcity in technical professionals. They are causing a great challenge for fast-tracking the maturity curve of an industry dedicated AI solutions.
Lack Of Information About AI
Artificial intelligence is there and is working out in giant strides. But still, there is a considerable lack of knowledge about AI and its requirement in real-time business environments. The manufacturing industry is facing a huge skill gap between the required functionalities and present-day high-end AI tools. Another hurdle is of successful mapping of use cases, which requires in-depth prior knowledge of AI and existing possible solutions.
AI has various uses, and the common ones are usually floating around the retail and customer side. Requirements of AI in the manufacturing industry require technical expertise which rarely found in common public domain.
Ignorance Of Pre-AI Development Research
One of the core prerequisite requirements of any industry-centric AI model is rigorous prior research. It is essential to analyze the existing processes, bring out conditions, and also change the company culture for data-driven practices. It is an integral part of any AI-powered solution adaption, and all manufacturing industries partially fail at it.
Picking the right processes for automation adoption requires technical expertise and data-driven analytics based on prior information and insights collected through performance-related data. Integrating all this information and building into relations is a tedious task, and it requires a consistent effort from the company and the AI solution development team.
Computational Ability
Majority of AI-powered solutions for various industries have ML algorithms and artificial neural networks underneath. Artificial neural networks were earlier away from general use because of their high-end computational abilities which were absent in the 20th century. Fine-tuning of the target variable through machine learning and natural capabilities is required for forecasting and predictive models.
It seeks next level computational power and the latest processors. It is a huge requirement that is not realized by industries that are actively looking to adopt such solutions. It becomes a significant reason for failure in the long run.
Cost Constraints
The budget overhead and financial burden created on any manufacturing businesses inevitable while adopting data-driven practices and AI-powered solutions. Please note that investments in artificial intelligence for manufacturing will never reap short-term benefits. They are investments from a long-term perspective that require a farsighted approach.
Every business has its own comfortable space and numbers. Higher profitability might be something there that is still on the milestone list. AI is the need of the present, but the cost constraints need to be respected for the overall sustainability of a business.
Fear Of Missing Out (FOMO) On Tech Solutions
Companies consider artificial intelligence in manufacturing industry or any other technology solution from a business perspective. It is crucial to analyze the proposition. It is incredibly essential not to get carried away by industry trends and finely propagated solutions. It’s important to weigh their benefits and how your business might fight the fear of missing out on the latest catchy technical solution.
Artificial intelligence solutions for manufacturing industries fall in the same category. The upfront focus should remain on cost feasibility and final projected business numbers after the successful adoption of an AI model. There is no point of going for any technological solution if your business doesn’t support its feasibility and maintenance in the long run.
Infrastructural Limitations
While walking through, we have established that successful adoption of artificial intelligence in manufacturing industries sector requires software, hardware, and technological infrastructure. It is essential to create a detailed checklist of the software, hardware, and present-day technical solutions available to your business at hand-stretch.
If a manufacturing business fails to successfully use any one (or more) of these requirements, you can expect unforeseen hurdles and deadlocks on your AI transformation journey.
Cost Feasibility In The Short Term
It is always advisable to look at profitability from a long-term perspective. But the reality says that short-term course feasibility cannot be ignored for successful survival of your business in competitive space.
Despite increasing costs due to technology, manufacturing businesses are getting much more competitive with their pricing models and ranges. To survive in a competitive environment, it is essential to see that incorporation of AI solutions does not put you out of the competition. You can expect increased survival costs and increasing and customer cost.
Conclusion
Artificial intelligence, a futuristic technology, and the manufacturing sector are bound to be impacted. Use of artificial intelligence-based practices in a manufacturing business is becoming a new dimension of competition, but it is imperative to analyze the prospects beforehand. It is always advisable to think the AI adoption journey throughout; right from a plan on paper to the results which you are most likely to achieve with the conservative approach.
It is always advisable to go for a financial consultancy before kick-starting any transformational adoptions of technology in your manufacturing business. Artificial intelligence is closely related to higher productivity and profitability for manufacturing, and it is going to impact the competition, criticality, and overall manufacturing space in the long run.
About The Author
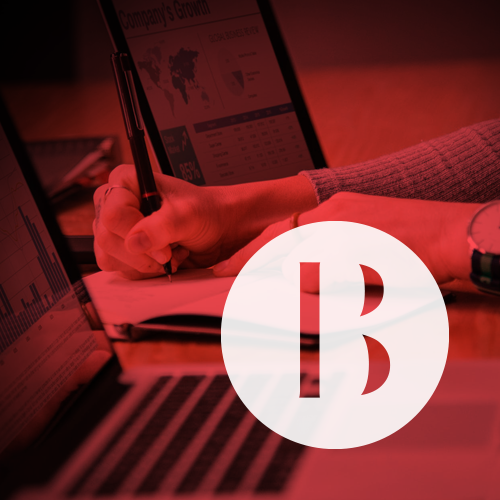